CU AI for Science Seminar AI for Cancer Research
>> YOUR LINK HERE: ___ http://youtube.com/watch?v=DzO_wMqgrMQ
The goal of the Cornell AI for Science Seminar Series is to bringing together the computational and scientific communities to understand advances and challenges at the frontier of AI for scientific discovery. In addition to senior speakers, the seminar features the research of Schmidt Futures AI for Science Postdoctoral researchers from multiple institutions. Seminars occur roughly biweekly on Fridays from 2:30-3:30pm EST. See our website for more information: https://science.ai.cornell.edu/ • We are excited to announce our first seminar featuring speakers from the Schmidt Futures AI in Science fellowship program! This week's seminar will feature Dr. Fatema Tuz Zohora and Dr. Xiaoyu Zhao on deep learning for cancer research! • AI Identifies Tumor Protein Complexes to Predict Chemoresistance • Dr. Xiaoyu Zhao, UCSD • Abstract: Understanding therapeutic resistance in cancer remains a longstanding challenge. Zhao and colleagues construct an interpretable AI system that integrates genetic alterations across molecular assemblies, enabling a quantitative and precise assessment of a tumor’s response to common chemotherapeutics that induce replication stress. The AI implicates 41 molecular assemblies involved in transcription, DNA repair, and cell-cycle regulation which underlie accurate drug response prediction. Thirty of these assemblies, which the authors further explore through CRISPR screenings, are found to regulate drug sensitivity and replication restart. The model translates to cisplatin-treated cervical cancer patients, revealing an RTK-JAK-STAT assembly linked to resistance. This research illuminates how a complex collection of mutations give rise to drug response, offering a valuable tool of interest for precision medicine. • Unraveling Cancer Cell Communication Using Deep Learning Models on Spatial Transcriptomics Data • Dr. Fatema Tuz Zohora, University of Toronto • Abstract: Cells coordinate within tissue to carry out complex functions, but dysregulation of communication between cells may lead to diseases such as cancer and diabetes. Identifying cell-cell communication that is upregulated in disease can lead to the development of better therapeutics. Existing computational methods for predicting communication in tumor samples often involve experiments that collect a single molecular layer from individual cells and do not take the spatial location of the cells into account. As a result, most existing methods exhibit high false positive rates. Moreover, most tools predict communication between cell populations instead of detecting communication at single-cell resolution, which misses the correlation between cellular communication and cancer heterogeneity. In this talk, I will present `NEST: NEural network on spatial transcriptomics', to address these limitations. NEST uses a graph attention network paired with an unsupervised contrastive learning approach to detect cellular communication while retaining the strength of each signal. NEST outperforms other methods on synthetic benchmarking experiments and detects biologically-relevant communication along with directionality and confidence across spot- and cell-based spatial transcriptomic technologies, tissues, and diseases. NEST identifies region-specific T-cell homing signals in human lymph nodes, aggressive cancer communication in lung adenocarcinoma, and discovers new communication patterns in pancreatic cancer. NEST is not limited to a single technology or species. Instead, it is a transferable model applicable to data across domains. We believe NEST makes a notable contribution to accelerating the application of deep learning in single-cell research and other related knowledge-graph based contexts.
#############################
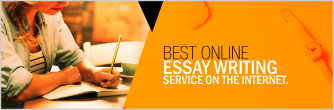