H2persistent homology from 3D image MRI of brain with glioblastoma
>> YOUR LINK HERE: ___ http://youtube.com/watch?v=fvhRbwnBFTo
arXiv link: https://arxiv.org/abs/2401.01160 • In our preprint Train-Free Segmentation in MRI with Cubical Persistent Homology , we adapt ideas from Topological Data Analysis to the problem of segmentation in medical images. This animation serves as an illustration of of persistent homology on a brain MRI. We build a cubical complex on the 3D greyscale image, with values between 0 and 1, and compute the persistent homology of its superlevel or sublevel sets filtration. The persistent homology computation is performed with the Python package Cubical Ripser https://github.com/shizuo-kaji/Cubica... • We analyse images from BraTS 2021, a collection of MRIs of brains with glioblastoma (tumour). The images come in two modalities: FLAIR and T1ce. In FLAIR, the tumour tends to be hyper-luminous, hence appear early in the superlevel sets filtrations. In T1ce, the Enhancing Tumour (tumour's contour) tends to be hypo-luminous, hence appear early in the sublevel sets filtration. For instance: • 0:09 - 0:35 - 1:08 The whole tumour is formed (FLAIR modality) • 0:25 - 0:53 - 1:25 Only the tumour's contour appears (T1ce modality) • At the core of our algorithm, we identify the whole tumour through the derivative of the curve of active voxels in the superlevel sets filtration of FLAIR (represented at the bottom right). It turns out that the tumour tends to appear just before the first significative peak of this curve. Besides, we estimate the Enhancing Tumour (tumour's contour) by selecting the most persistent H2-cycle of the sublevel sets filtration of T1ce. Indeed, the contour tends to form a sphere, hence is represented by a highly persistent point in the persistence diagram (represented at the top right). • Abstract: We describe a new general method for segmentation in MRI scans using Topological Data Analysis (TDA), offering several advantages over traditional machine learning approaches. • It works in three steps, first identifying the whole object to segment via automatic thresholding, then detecting a distinctive subset whose topology is known in advance, and finally deducing the various components of the segmentation. Although convoking classical ideas of TDA, such an algorithm has never been proposed separately from deep learning methods. To achieve this, our approach takes into account, in addition to the homology of the image, the localization of representative cycles, a piece of information that seems never to have been exploited in this context. In particular, it offers the ability to perform segmentation without the need for large annotated data sets. TDA also provides a more interpretable and stable framework for segmentation by explicitly mapping topological features to segmentation components. By adapting the geometric object to be detected, the algorithm can be adjusted to a wide range of data segmentation challenges. We carefully study the examples of glioblastoma segmentation in brain MRI, where a sphere is to be detected, as well as myocardium in cardiac MRI, involving a cylinder, and cortical plate detection in fetal brain MRI, whose 2D slices are circles. We compare our method to state-of-the-art algorithms.
#############################
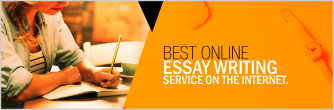