Assumptions of Linear Regression
>> YOUR LINK HERE: ___ http://youtube.com/watch?v=sDrAoR17pNM
Assumptions of Linear Regression: In order for the results of the regression analysis to be interpreted meaningfully, certain conditions must be met: • 1) Linearity: There must be a linear relationship between the dependent and independent variables. • 2) Homoscedasticity: The residuals must have a constant variance. • 3) Normality: The residuals must be normally distributed. • 4) No Multicollinearity: No high correlation between the independent variables • Linearity: • In linear regression, a straight line is placed through the data. This straight line should represent all points as good as possible. If the relation is nonlinear the straight line cannot fulfill this requirement. • Normal distribution of the error: • One assumption of linear Regression is that the error epsilon must be normally distributed, • To check this there are two ways, one is the analytical way and the other is the graphical way. • Homoscedasticity: • A assumption for linear regression is that the residuals have a constant variance. • Since your regression model never exactly predicts your dependent variable in practice, you always have an error. Now you can plot your dependent variable on the x axis and the error on the y axis. • Multicollinearity: • In multicollinearity, two or more of the predictors correlate strongly with each other. • Test your assumptions for the linear Regression online: • https://datatab.net/statistics-calcul... • And here are mor informations about Regression: • https://datatab.net/tutorial/linear-r...
#############################
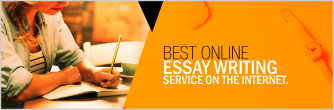