The Evolution of Translation Efficiency IMUG 20240320
>> YOUR LINK HERE: ___ http://youtube.com/watch?v=ZISYtTVoa_Y
Index: • 0:00 Intro - Speaker - Adam Bittlingmayer, CEO of ModelFront, ex-Google Translate engineer, language guy • 0:58 Problem: We translate almost nothing. • 3:04 Debug: Why don't we translate more? • 8:13 Progress metric: How many segments are perfect (unedited)? • 11:37 Solution: Machine translation quality prediction • 14:10 Next steps: How to get there? • 24:52 (Q A begins) • 25:18 - Accelerating real-time speech translation? • 27:52 - Generate directly in target languages? No translation. • 34:50 - When will machines surpass humans? • 36:31 - Tracking human translator edits? • 37:50 - What about tracking human translator time? • 39:30 - How to evaluate machine translation quality prediction? A/B testing • 40:30 - Generating and predicting tone vs accuracy? • 41:54 - Generate directly in target languages? No translation. Part II. • 48:02 - How to make concrete forward progress? • 50:21 - Censorship and political correctness in model guardrails • 52:45 - What can stop progress? Data rights? Data contamination? • 55:47 - A/B testing example? • 58:33 - Metadata and context as model input? Limitations of major cloud MT APIs? • Topic description: • :: Levels of efficiency for human-quality translation workflows :: • What's the next level of translation efficiency? Why is post-editing machine translation not that much faster, and what is? What are the prerequisites for using more AI successfully? And what about quality? • We'll walk through the levels of efficiency for human-quality translation workflows, from fully manual, to the hybrid workflows used to translate tens or hundreds of millions of words, whether inside ecom platforms and enterprise L10n buyer teams, like Microsoft, Citrix and VMWare, or LSPs with tech startup DNA, like Unbabel. • We'll answer practical questions about savings, maintaining quality, adoption, prerequisites, availability in translation management systems (TMSes) and costs. • “The future is already here. It's just not evenly distributed.” • Starting in 2013, leading players researched and launched AI beyond MT - like adaptive translation, quality prediction or automatic post-editing. • But for L10n teams, getting AI beyond MT wasn't in reach without in-house machine learning research team, and wasn't accessible in TMSes anyway. • So by 2020, much of the industry - even L10n teams inside Bay Area tech companies - was still stuck post-editing generic machine translation, or not even post-editing at all. • Now that has changed, thanks to providers of AI for adaptive translation and quality prediction - integrated by the top TMSes - as well as tools like ChatGPT, that let anybody play with an LLM for many more tasks. • About the speaker: • Adam Bittlingmayer is the CEO and co-founder of ModelFront, the leading provider of machine translation quality prediction. • To translate their content more efficiently - at the same human quality - high-volume translation buyers use segment-level quality scores to control which segments get sent to human editing, and which don't - because they won't be edited anyway. • Adam previously worked at Google Translate as a software engineer, as well as on products like Android Market (Google Play) and Adobe Creative Suite. • He also founded the non-profit Machine Translate foundation to make machine translation more accessible to more people, with open information and community. machinetranslate.org now covers 75 APIs, 251 TMS integrations and 225 languages.
#############################
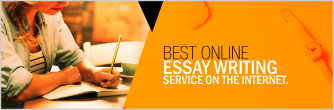